This new data poisoning tool lets artists fight back against generative AI
The tool, called Nightshade, messes up training data in ways that could cause serious damage to image-generating AI models.

A new tool lets artists add invisible changes to the pixels in their art before they upload it online so that if it’s scraped into an AI training set, it can cause the resulting model to break in chaotic and unpredictable ways.
The tool, called Nightshade, is intended as a way to fight back against AI companies that use artists’ work to train their models without the creator’s permission. Using it to “poison” this training data could damage future iterations of image-generating AI models, such as DALL-E, Midjourney, and Stable Diffusion, by rendering some of their outputs useless—dogs become cats, cars become cows, and so forth. MIT Technology Review got an exclusive preview of the research, which has been submitted for peer review at computer security conference Usenix.
AI companies such as OpenAI, Meta, Google, and Stability AI are facing a slew of lawsuits from artists who claim that their copyrighted material and personal information was scraped without consent or compensation. Ben Zhao, a professor at the University of Chicago, who led the team that created Nightshade, says the hope is that it will help tip the power balance back from AI companies towards artists, by creating a powerful deterrent against disrespecting artists’ copyright and intellectual property. Meta, Google, Stability AI, and OpenAI did not respond to MIT Technology Review’s request for comment on how they might respond.
Zhao’s team also developed Glaze, a tool that allows artists to “mask” their own personal style to prevent it from being scraped by AI companies. It works in a similar way to Nightshade: by changing the pixels of images in subtle ways that are invisible to the human eye but manipulate machine-learning models to interpret the image as something different from what it actually shows.
The team intends to integrate Nightshade into Glaze, and artists can choose whether they want to use the data-poisoning tool or not. The team is also making Nightshade open source, which would allow others to tinker with it and make their own versions. The more people use it and make their own versions of it, the more powerful the tool becomes, Zhao says. The data sets for large AI models can consist of billions of images, so the more poisoned images can be scraped into the model, the more damage the technique will cause.
A targeted attack
Nightshade exploits a security vulnerability in generative AI models, one arising from the fact that they are trained on vast amounts of data—in this case, images that have been hoovered from the internet. Nightshade messes with those images.
Artists who want to upload their work online but don’t want their images to be scraped by AI companies can upload them to Glaze and choose to mask it with an art style different from theirs. They can then also opt to use Nightshade. Once AI developers scrape the internet to get more data to tweak an existing AI model or build a new one, these poisoned samples make their way into the model’s data set and cause it to malfunction.
Poisoned data samples can manipulate models into learning, for example, that images of hats are cakes, and images of handbags are toasters. The poisoned data is very difficult to remove, as it requires tech companies to painstakingly find and delete each corrupted sample.
The researchers tested the attack on Stable Diffusion’s latest models and on an AI model they trained themselves from scratch. When they fed Stable Diffusion just 50 poisoned images of dogs and then prompted it to create images of dogs itself, the output started looking weird—creatures with too many limbs and cartoonish faces. With 300 poisoned samples, an attacker can manipulate Stable Diffusion to generate images of dogs to look like cats.
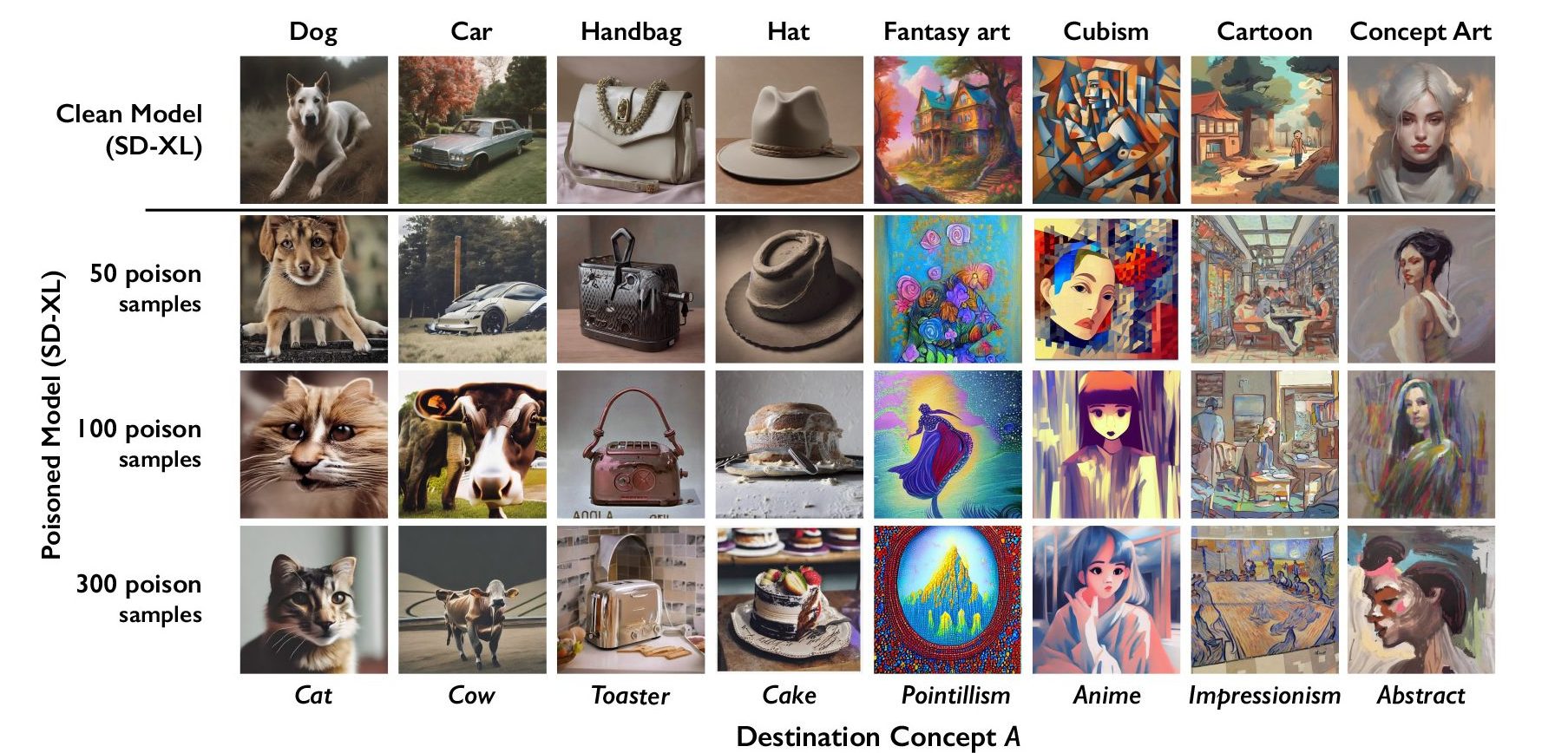
Generative AI models are excellent at making connections between words, which helps the poison spread. Nightshade infects not only the word “dog” but all similar concepts, such as “puppy,” “husky,” and “wolf.” The poison attack also works on tangentially related images. For example, if the model scraped a poisoned image for the prompt “fantasy art,” the prompts “dragon” and “a castle in The Lord of the Rings” would similarly be manipulated into something else.

Zhao admits there is a risk that people might abuse the data poisoning technique for malicious uses. However, he says attackers would need thousands of poisoned samples to inflict real damage on larger, more powerful models, as they are trained on billions of data samples.
“We don’t yet know of robust defenses against these attacks. We haven’t yet seen poisoning attacks on modern [machine learning] models in the wild, but it could be just a matter of time,” says Vitaly Shmatikov, a professor at Cornell University who studies AI model security and was not involved in the research. “The time to work on defenses is now,” Shmatikov adds.
Gautam Kamath, an assistant professor at the University of Waterloo who researches data privacy and robustness in AI models and wasn’t involved in the study, says the work is “fantastic.”
The research shows that vulnerabilities “don’t magically go away for these new models, and in fact only become more serious,” Kamath says. “This is especially true as these models become more powerful and people place more trust in them, since the stakes only rise over time.”
A powerful deterrent
Junfeng Yang, a computer science professor at Columbia University, who has studied the security of deep-learning systems and wasn’t involved in the work, says Nightshade could have a big impact if it makes AI companies respect artists’ rights more—for example, by being more willing to pay out royalties.
AI companies that have developed generative text-to-image models, such as Stability AI and OpenAI, have offered to let artists opt out of having their images used to train future versions of the models. But artists say this is not enough. Eva Toorenent, an illustrator and artist who has used Glaze, says opt-out policies require artists to jump through hoops and still leave tech companies with all the power.
Toorenent hopes Nightshade will change the status quo.
“It is going to make [AI companies] think twice, because they have the possibility of destroying their entire model by taking our work without our consent,” she says.
Autumn Beverly, another artist, says tools like Nightshade and Glaze have given her the confidence to post her work online again. She previously removed it from the internet after discovering it had been scraped without her consent into the popular LAION image database.
“I’m just really grateful that we have a tool that can help return the power back to the artists for their own work,” she says.
Deep Dive
Artificial intelligence
Large language models can do jaw-dropping things. But nobody knows exactly why.
And that's a problem. Figuring it out is one of the biggest scientific puzzles of our time and a crucial step towards controlling more powerful future models.
OpenAI teases an amazing new generative video model called Sora
The firm is sharing Sora with a small group of safety testers but the rest of us will have to wait to learn more.
Google DeepMind’s new generative model makes Super Mario–like games from scratch
Genie learns how to control games by watching hours and hours of video. It could help train next-gen robots too.
Responsible technology use in the AI age
AI presents distinct social and ethical challenges, but its sudden rise presents a singular opportunity for responsible adoption.
Stay connected
Get the latest updates from
MIT Technology Review
Discover special offers, top stories, upcoming events, and more.